Revolutionizing Machine Learning with Video Annotation Tools
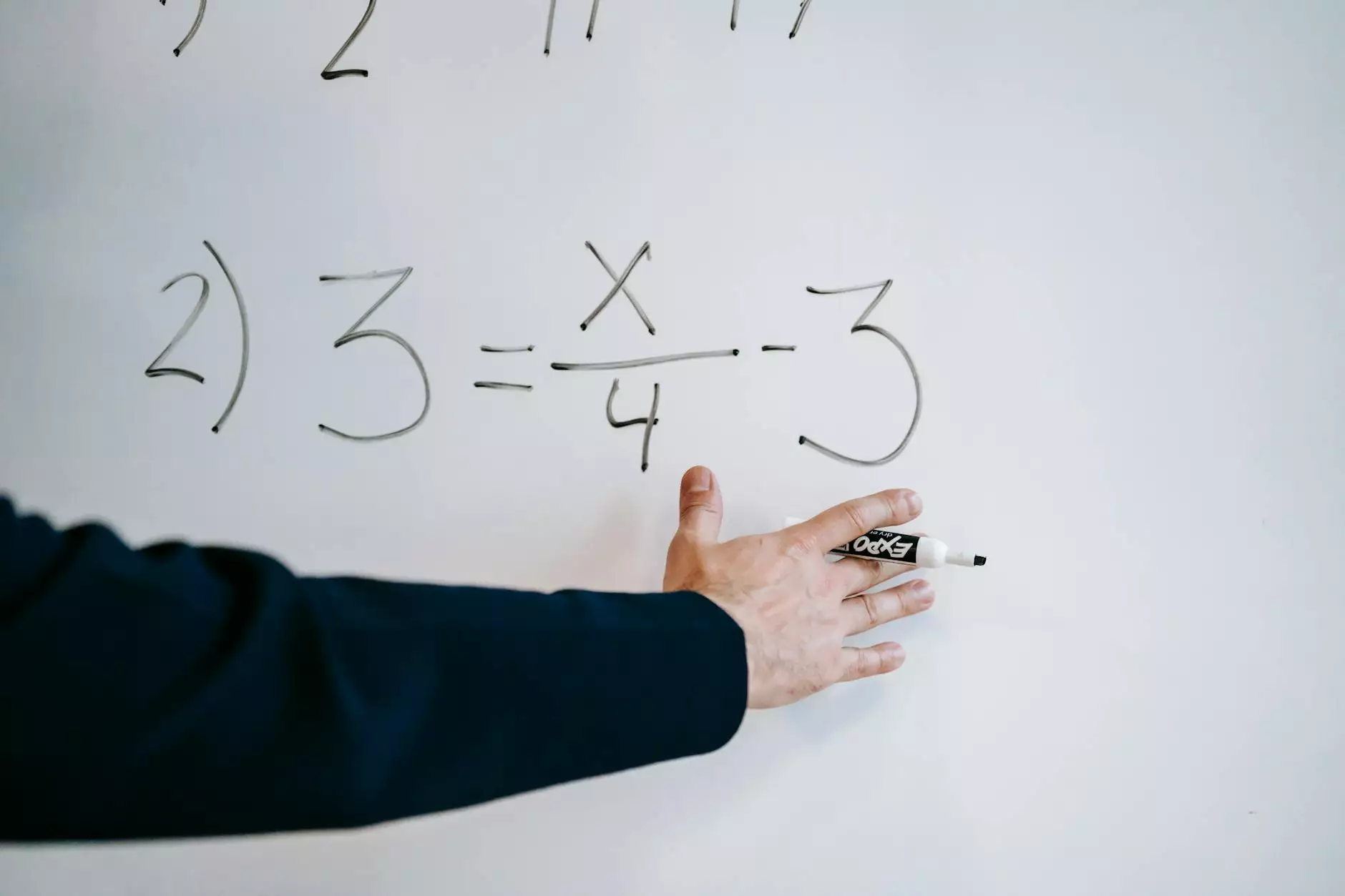
Understanding the Importance of Video Annotation in Machine Learning
In today’s world, machine learning (ML) has emerged as a transformative technology across various industries. One crucial aspect of machine learning is the availability and quality of training data. With the rapid advancement of technology, video data has become an incredibly valuable resource for training algorithms, particularly in fields such as computer vision, autonomous driving, and facial recognition.
As the volume of video data increases, so does the need for sophisticated tools to aid in processing and annotating this data efficiently. This is where a video annotation tool for machine learning becomes essential. These tools enable users to label and categorize video content, which is fundamental for the model's performance in predicting outcomes based on new data.
What is Video Annotation?
Video annotation refers to the process of adding notes, labels, or other identifiers to video content. This helps in creating annotations that describe specific features or actions within the video. This information is crucial for training machine learning models because it provides context to raw video data.
Types of Video Annotations
- Object Detection: Labeling objects within each frame of the video.
- Action Recognition: Annotating movements or actions performed by subjects in the video.
- Semantic Segmentation: Marking pixel-level annotations to distinguish between different objects and backgrounds.
- Scene Recognition: Classifying various scenes depicted in the video.
The Role of Video Annotation Tools in Machine Learning
With the increasing complexity of machine learning models, having the right tools to create quality training datasets is more important than ever. A video annotation tool for machine learning simplifies the process of annotating data through a user-friendly interface and often includes several advanced features, such as:
1. Automated Annotation Features
Many modern tools incorporate artificial intelligence (AI) to suggest or even automate annotations based on previously labeled data. This can significantly speed up the annotation process and ensure consistency across datasets.
2. Multi-User Collaboration
A robust video annotation tool allows multiple users to collaborate in real-time, making it easy for teams to work together on large datasets. This collaborative approach increases productivity and ensures a diverse range of inputs when labeling data.
3. Customizable Annotation Formats
Different projects might require different types of annotations. A versatile tool provides options for customizing annotation formats to suit specific project needs, whether it's bounding boxes for object detection or more complex segmentation options.
Why Choose a Video Annotation Tool for Your Business?
For businesses involved in machine learning, investing in a solid video annotation tool is critical for several reasons:
1. Enhanced Data Quality
Quality data leads to better machine learning models. Accurate video annotations significantly enhance the quality of training datasets, which directly impacts model performance.
2. Increased Efficiency
With automated features and collaborative capabilities, these tools streamline the annotation process, allowing teams to focus more on analysis and less on manual data entry.
3. Scalability
As your business grows, so will your data needs. A scalable annotation tool can adapt to handle increasing amounts of data without compromising on quality or turnaround times.
4. Competitive Advantage
In industries where data is king, having superior annotated datasets can offer a competitive edge, allowing businesses to develop more accurate models faster than their competitors.
Key Industries Utilizing Video Annotation Tools
The applications of video annotation tools are vast and varied. Here are some key industries benefiting from their use:
1. Automotive
In the automotive industry, video annotation plays a crucial role in developing autonomous driving systems. Annotated driving data helps train vehicles to recognize pedestrians, traffic signs, and various driving conditions.
2. Retail
Retail businesses use video annotation for analyzing shopping behaviors. By understanding how customers interact with products, companies can improve store layouts and product placements.
3. Security and Surveillance
Security firms utilize annotated video data for building systems that enhance real-time monitoring and threat detection. Accurate labeling of behaviors helps in training systems to recognize suspicious activities.
4. Healthcare
In healthcare, video annotations are essential for analyzing medical procedures and training AI systems to assist doctors in diagnosis and treatment planning.
Choosing the Right Video Annotation Tool
When selecting a video annotation tool for machine learning, consider the following factors:
1. User-Friendliness
The interface should be intuitive, allowing users to quickly learn how to utilize all features effectively without extensive training.
2. Feature Set
Look for tools that offer a robust set of features, including automated annotation, support for various formats, and collaboration capabilities. This diversity will help adapt the tool to your specific needs.
3. Support and Resources
Reliable customer support and comprehensive documentation can help resolve issues quickly and ease the learning process for new users.
4. Integration Capabilities
The tool should easily integrate with other platforms you may be using, such as cloud storage solutions or machine learning frameworks.
Future Trends in Video Annotation Tools
As technology continues to advance, we can expect several trends in video annotation tools:
1. Increased Use of Deep Learning
A deep learning approach to video annotation could significantly improve automation and accuracy, allowing tools to learn from user inputs over time.
2. Enhanced User Interfaces
Future tools will likely feature more sophisticated user interfaces with better visualization tools, making the process of annotating videos even easier for users.
3. Integration of Virtual and Augmented Reality
Incorporating VR and AR technologies could allow for more interactive methods of annotation, leading to improved understanding and better-trained models.
4. Growing Importance of Ethics in AI
As machine learning continues to evolve, ethical considerations in data annotation will become increasingly important. Ensuring that data is collected and annotated in a manner that mitigates bias and promotes fairness will be critical.
Conclusion
In conclusion, choosing the best video annotation tool for machine learning is vital for businesses aiming to leverage the power of video data. These tools not only improve data quality and efficiency but also provide a competitive edge in a rapidly advancing technological landscape. Whether you are in the automotive, retail, security, or healthcare industry, leveraging high-quality annotated video data can significantly enhance your machine learning models and outcomes.
Investing in a superior video annotation tool is a step towards ensuring your business remains relevant and thrives in the competitive market, paving the way for innovations that can shape the future of your industry.